AI in the public sector: from black boxes to meaningful transparency
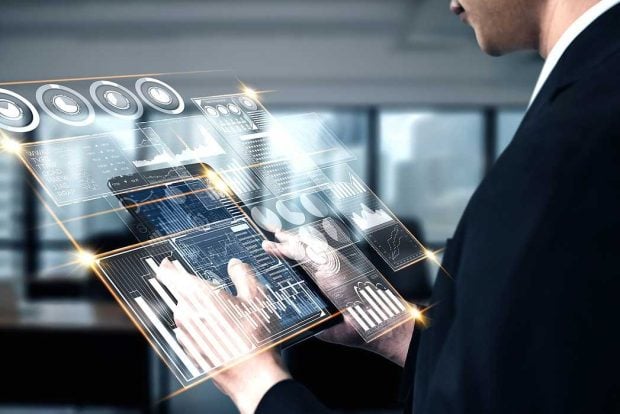
Getting transparency right around AI isn’t just good for democracy, it’s good for the public sector too, writes Imogen Parker, associate director at the Ada Lovelace Institute
Within AI research there is a lot of discussion about ‘black box’ systems and the difficulty of knowing or understanding how or why some AI systems behave the way they do. Addressing this challenge is becoming more important as AI tools become even more technically complex and commonplace.
When it comes to public services, we face an analogous challenge that moves from the technical to the social. We know very little about how or where AI tools are being used in the public sector. The use of AI in the public sector risks becoming a ‘black box’ itself.
Our nationally representative public attitudes survey with the Alan Turing Institute found public awareness is rising for more visible AI uses, like facial recognition for border control. However, we are much less aware when AI is used as part of wider government systems like the prioritisation of housing or assessment of welfare benefits.
In the UK, I doubt anyone reading this will be aware of, or able to even find out, whether their child is being risk assessed based on an analysis of education data, household finances and family structures. Or whether the local police force is using data science to predict their likelihood of being in a gang. Or whether information about a separation or divorce is being used to predict risk of future debt or homelessness.
While some specific cases have garnered individual attention as they hit the headlines, there remains a persistent, systemic deficit in public understanding about how and where AI systems are being used to make important and high-stakes decisions.
Ironically, while these systems are often invisible to the public, people are becoming ever more visible to public services through ‘datafication’, with services using that data to make decisions about them, their families and their services.
Read more: AI in public services – a political panacea?
Algorithmic transparency
In response to these challenges the UK government developed the ‘Algorithmic Transparency Recording Standard’ (ATRS) in 2021. The aim was to support meaningful transparency into algorithm-assisted decisions by encouraging organisations to publish details about their algorithmic tools.
It was an exciting milestone – and one we’d both called for and worked with the government to design. At the time we felt that much of the debate jumped into the rights or wrongs of different uses of AI without any actual baseline understanding of what AI was being used where and for what purpose.
Despite the creation of an expanded hub for the standard in 2023, and an announcement from the UK’s previous government that the ATRS would be a requirement for all central government departments, the repository of completed records still stands at only nine entries. We know work has been going on to map the use of algorithmic decision-making in the public sector, but we have yet to see this published.
Transparency is easier said than done: even for those working within the sector, complex systems, arcane procurement procedures, commercial sensitivities, impenetrable legalese and obtuse jargon from both providers and public services all combine to make it very difficult to understand exactly how data-driven technologies are being used.
This leaves AI in the public sector with a ‘black box’ problem that is social rather than technical. While that poses an issue in terms of democratic accountability and public understanding, our research with public servants and public sector workers also highlights where a lack of transparency limits their successful adoption and use.
Under the bonnet
On the rare occasions where we have been able to get under the bonnet of AI and data-driven systems in the public sector, transparency issues have had an impact on the confidence and trust of frontline workers in using AI tools.
For example, at the Ada Lovelace Institute we conducted a research project exploring the use of privately provided data analytics in the context of children’s social care and social support in response to the COVID-19 pandemic. The system would flag higher risk families to frontline workers, based on its own predictive model.
Some categories used as risk factors by the model were expected – like child exploitation, sexual abuse and substance misuse. But frontline workers also described the use of vague categories (“general concerns”) and highly value-laden categories like “undesirable behaviour” (separate to “criminality”) without any clarity about what these were and how and why they were being applied.
As well as creating a legitimacy issue (residents were unaware this tool was being used) this understanding gap undermined the potential benefits of the technology. Frontline workers were less comfortable using the insights or recommendations provided because they couldn’t explain them or felt they wouldn’t be seen as legitimately acquired:
As one said: “[If an algorithm] identifies the family, and we pick up the phone, what do we actually say to them?… Nothing has actually happened to this family. There’s been no incident.”
The key regulator here – the Information Commissioner’s Office – has set the bar high for what good explainability ought to look like for these types of predictive or decision-making tools. But this is only good practice guidance rather than a legal requirement or enforced standard.
Read more: Making AI work for people and society
Procurement
Speaking to those procuring AI across the public sector has also highlighted another issue hindering good outcomes: a lack of transparency limits coordination and the ability to learn lessons about what works and what doesn’t.
Individually and collectively, we are a long way away from being able to scrutinise and evaluate the impact of public sector AI on communities and individuals. And there is no systematic approach to this type of evaluation at the national level. I’ve been in countless meetings where different parts of the government have only found out about particular pilots or deployments by chance through being in meetings or workshops with colleagues.
While some AI tools on offer lack scientific backing, such as AI systems attempting to identify a person’s sexuality or emotions from facial data, others might offer real opportunities to transform services, like freeing up police officers by automating repetitive paperwork. But our understanding of public sector AI is still far too reliant on the sales pitches of private companies. These tools are being sold to the public sector and rolled out piecemeal without sufficiently joined-up oversight or accountability.
Meaningful transparency is therefore urgently needed – both to support public understanding of tools that might affect them, but also to support the beneficial adoption and evaluation of novel AI tools. Getting transparency right is a prerequisite to responsible use of AI in the public interest and mitigating the potential harms from inappropriate or flawed uses of AI.